-
7 mei
-
Highlighted
'Every three months, AI's energy consumption doubles'
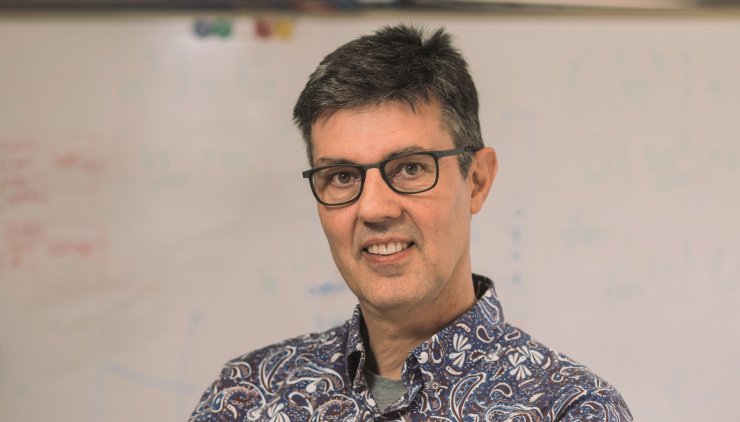
Artificial Intelligence: you see it around you more and more. But despite all the success stories, there is a dark side to it, says Gerard Schouten. He is a lecturer in AI and big data at Fontys ICT and affiliated with the Knowledge Center Applied AI for Society. 'Those AI models cost a lot of energy. That is why, among other things, we are studying Green AI: how to make AI more sustainable?’
Sustainability of AI is a still under-explored issue, Gerard argues. 'A lot of attention is paid to the human-centered view of AI, such as the ethical issues. But how to reduce the carbon footprint of AI is still a fairly new field. With the trend of AI models getting bigger and more complex, it's important to draw more attention to that.
Gerard gives an example from one of the earlier versions of ChatGPT. 'First, the AI model had to be trained with data. That required time and computing power from computers. The amount of energy required for that was equivalent to 500 tons of CO2. That's as much as the energy consumption of a thousand cars traveling a thousand kilometers each.' And using ChatGPT also consumes a lot of power. 'A single question-answer dialogue can be compared to the amount of energy needed to charge your phone. Much more than when you use search engine Google. And every day there are millions of these kinds of mini-conversations with the chatbot ChatGPT.
Energy-efficient AI models
According to Gerard, every three months the energy consumption consumed by AI doubles. To make AI models more energy efficient, Gerard and colleague Qin Zhao have formulated five tips. Consider, for example, using tools that measure how much energy an AI model consumes. 'We tell students: monitor and report energy consumption. With green or local energy, for example, you have a lower carbon footprint. So don't just build an AI model that is result-oriented, but also state how much energy it costs.'
Furthermore, Gerard recommends choosing a simpler AI base model sooner. Software developers tend to grab the biggest and most energy-guzzling base models already available in open-source tools. But often you can already achieve the desired results with a simpler AI model.' And also try to limit re-training your AI model. 'Don't start doing that immediately when you have new data, but look carefully at when it really matters.' There is also much to be gained from optimizing data quality and training schedules for the AI model.
Energy transition
So despite the fact that AI models are consuming more and more energy, there are also positive sides to the use of AI. For it can actually be used to reduce energy consumption. 'Nowadays at the energy grid you have not only producers and consumers, but also prosumers. People who are both, for example, when you have solar panels on your roof. But what do you do when the sun isn't shining and you do want a charged electric car? AI and data solutions such as apps can help shape the energy transition smarter in such a dynamic prosumer scenario. For example, by coordinating with the energy network when you want to store excess generated energy. So that you can use that energy at a later time for charging your car or for other devices.'
Right now, AI provides even more sustainability than it costs energy. 'Fine,' says Gerard, 'but how can we reduce that consumption? That starts with awareness. Ideally, we want to introduce the basic principles to reduce the energy consumption of AI at all colleges and universities. That way the new generation of professionals can make reliable and green AI models.'